An Agent Based Model of Environmental Awareness and Limited Resource Consumption
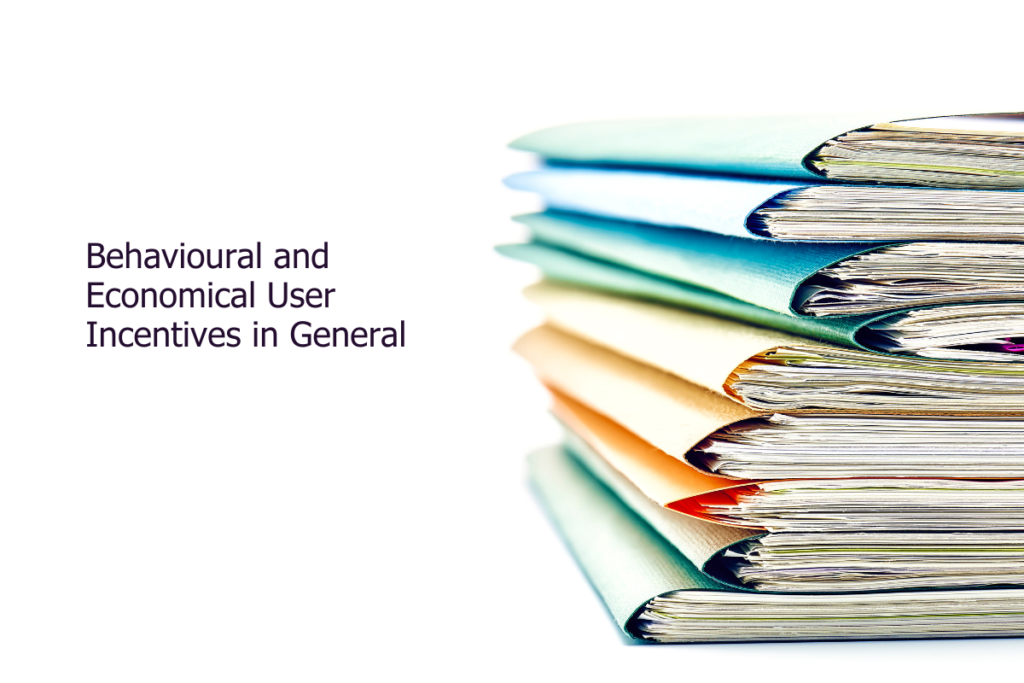
Scope Damiani & Sissa introduce an Agent-Based Model that explores how environmental awareness spreads within a community and influences resource consumption behaviors, such as energy or water use. Summary The model features five types of agents that range from resistant “blinds” to activists referred to as “evangelists”. It examines how the agents’ interactions shape overall community […]
An Awareness Based Approach to Avoid Rebound Effects in ICTs
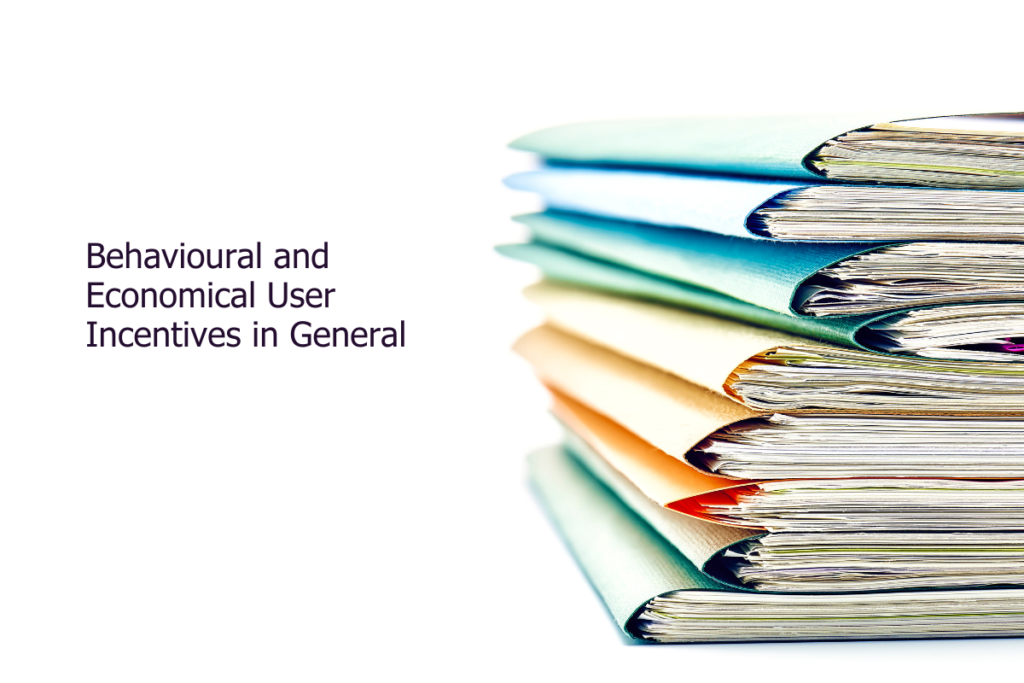
Scope Sissa discusses the social dimension towards the full exploitation of ICT-related environmental benefits, focusing on the role that users, consumers or citizens can play in spreading and adopting pro-environmental ICT behaviour, answering to the question why incentives are important. Precisely, the active participative role of consumers promotes the collective situational awareness about environmental effects of […]
Reconsidering CO2 Emissions from Computer Vision
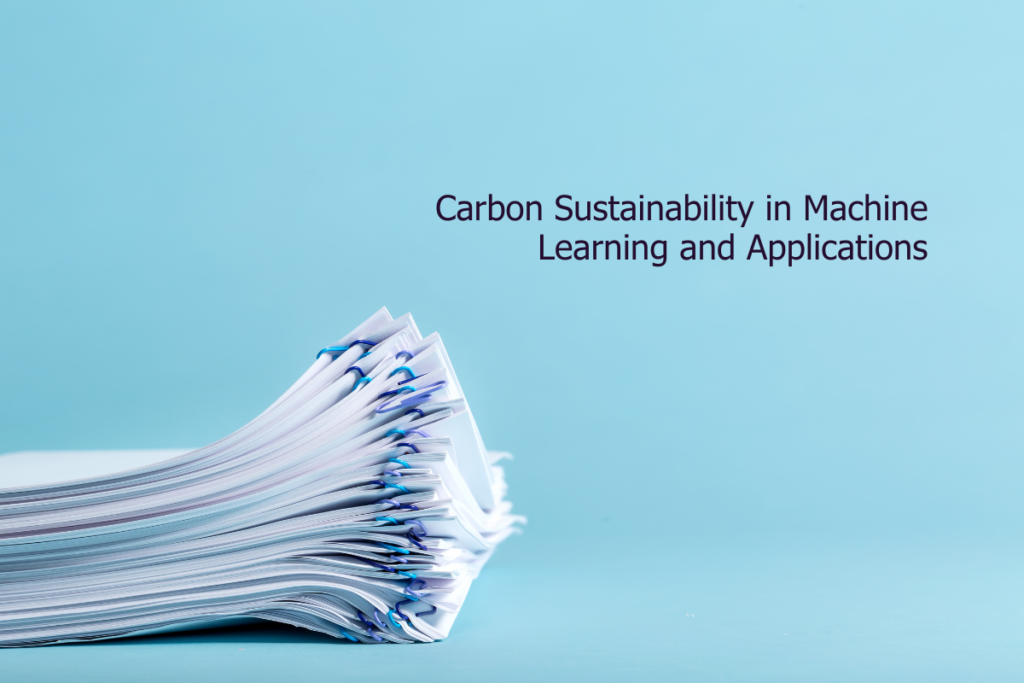
Scope In (Fu et al, 2021) the authors investigate the carbon footprint of Computer Vision methodologies by decomposing the total CO2 emissions into two components: the emissions incurred during the initial model development phase and those accumulated during the model’s lifetime evaluation (training and usage). The paper provides quantitative metrics based on GPU hours, FLOPS, […]
Towards Energy Consumption and Carbon Footprint Testing for AI-driven IoT Services
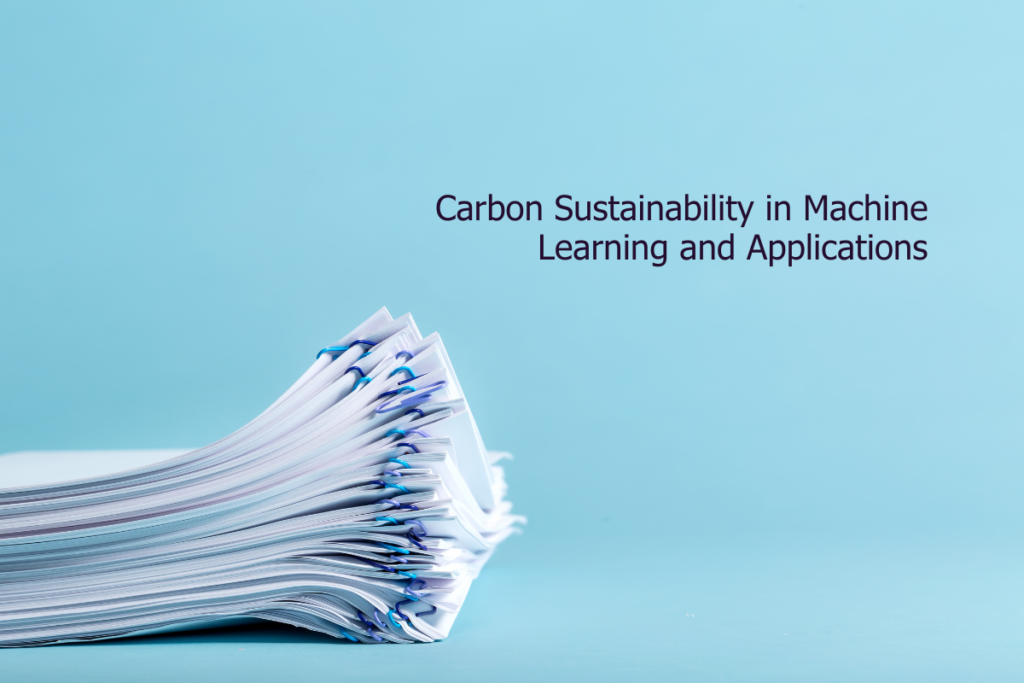
Scope In (Trihinas et al, 2022) the authors provide a comprehensive overview of the metrics and methodologies required to estimate energy consumption and carbon emissions in IoT settings. It covers: i) fundamental concepts such as power consumption (using equations for energy, power usage effectiveness, etc.) and carbon intensity, ii) experimental evaluation of AI-driven IoT services […]
Achieving Net Zero Emissions with Machine Learning: The Challenge Ahead
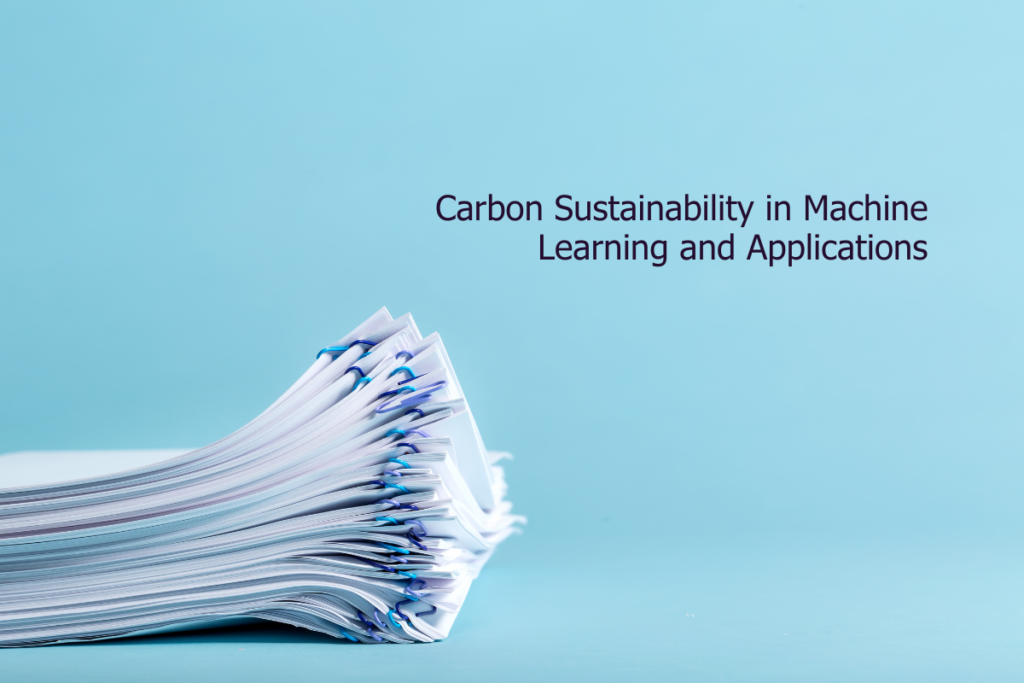
Scope The article (Nature Editorial, 2022) emphasizes the urgency of limiting global warming to 1.5C, citing the Intergovernmental Panel on Climate Change (IPCC) report which stresses the need for global greenhouse gas emissions to peak by 2025 and to fall by 43% by 2030. The editorial explores several areas where ML can contribute to this […]
Cryptocarbon: How Much is the Corrective Tax?
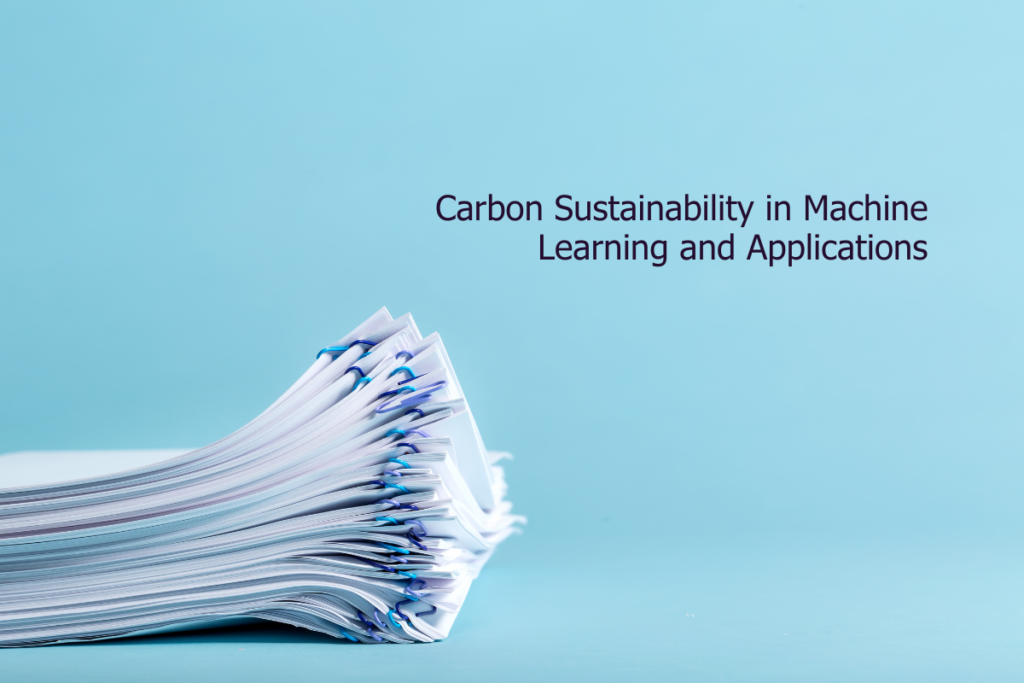
Scope In (Hebous and Vernon-Lin, 2024) explores the environmental impact of cryptocurrency mining, particularly the carbon emissions resulting from the energy-intensive proof-of-work protocol. For that, the authors introduce the concept of cryptocarbon to quantify the carbon emissions caused by the cryptomining activities. They focus on estimating the sufficient corrective tax to address the negative externalities […]
Training a Single AI Model Can Emit As Much Carbon As Five Cars in Their Lifetimes
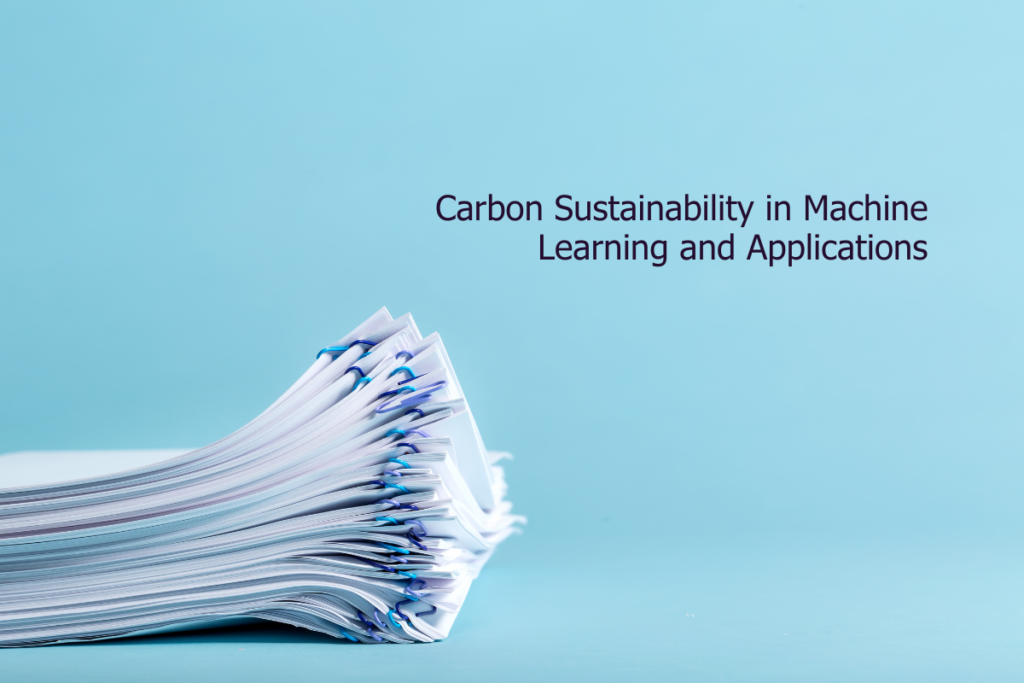
Scope In (Hao, 2019) the author explores the environmental impact of training large artificial intelligence (AI) models, particularly in the field of natural language processing (NLP). Summary AI’s carbon footprint is a growing concern, as training a single AI model can generate as much CO2 as the lifetime emissions of five cars. Research from the […]
Aligning Artificial Intelligence with Climate Change Mitigation
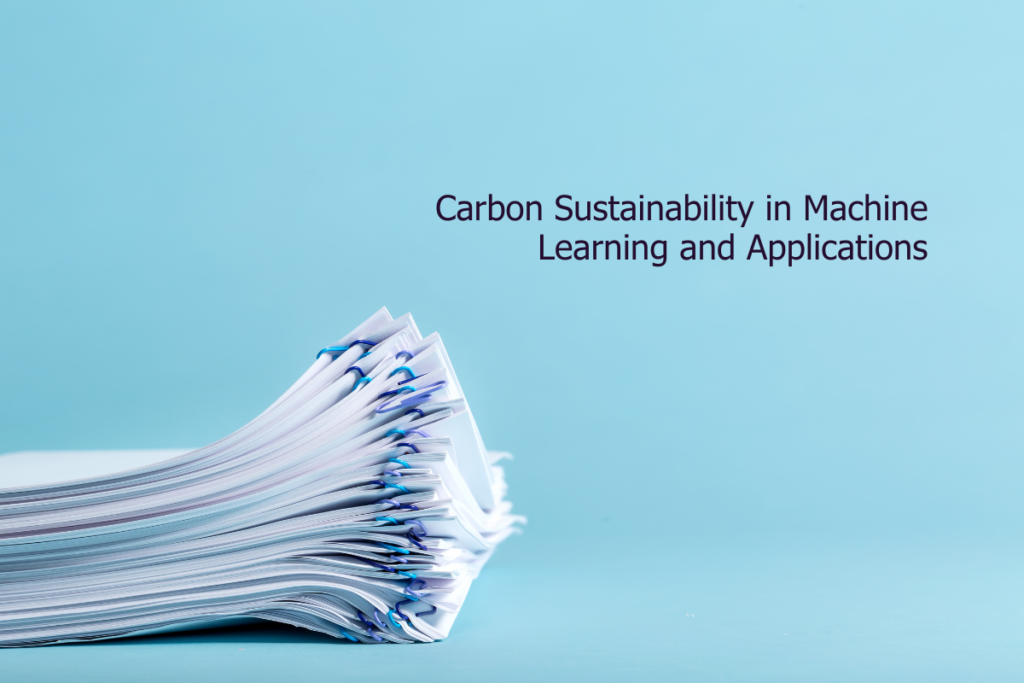
Scope In (Kaack et al, 2022) provides a systematic framework for analysing the relationship between ML and climate change mitigation. The authors classify the impact of ML on greenhouse gas GHG emissions into three categories: (A) compute-related emissions from hardware and energy use, (B) emissions from the immediate applications of ML, and (C) system-level impacts, […]
Counting Carbon: A Survey of Factors Influencing the Emissions of Machine Learning
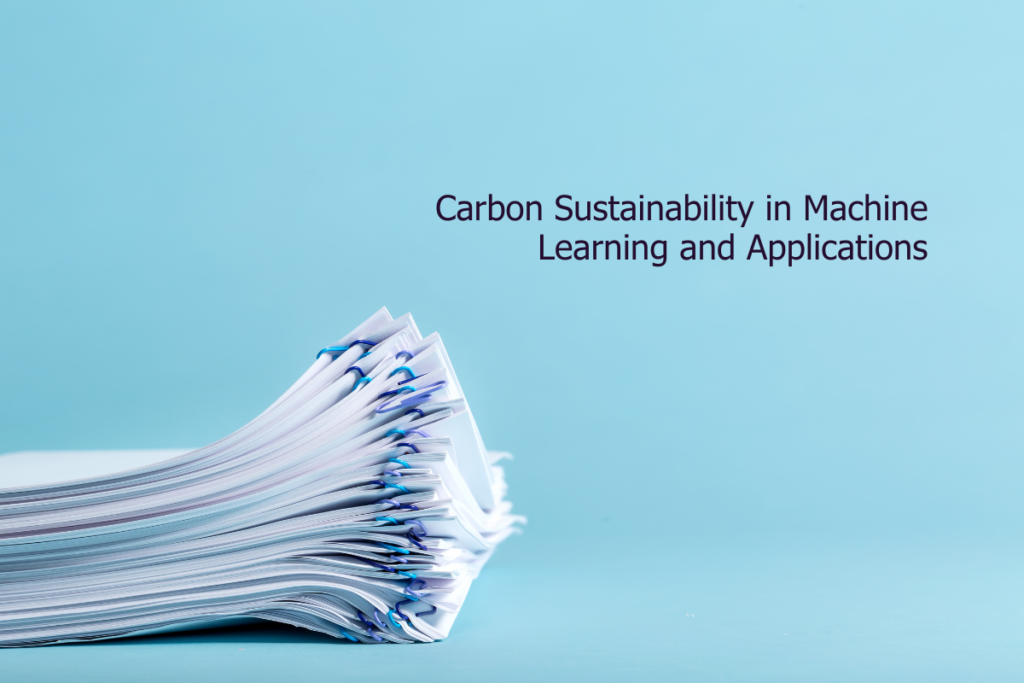
Scope In (Luccioni et al, 2023) the authors provide a comprehensive survey of the factors that contribute to the carbon emissions of machine learning (ML) models. The authors highlight the energy consumption required to develop and deploy ML models, focusing on how different models and tasks generate varying levels of greenhouse gas emissions. The study […]
Carbon Emissions and Large Neural Network Training
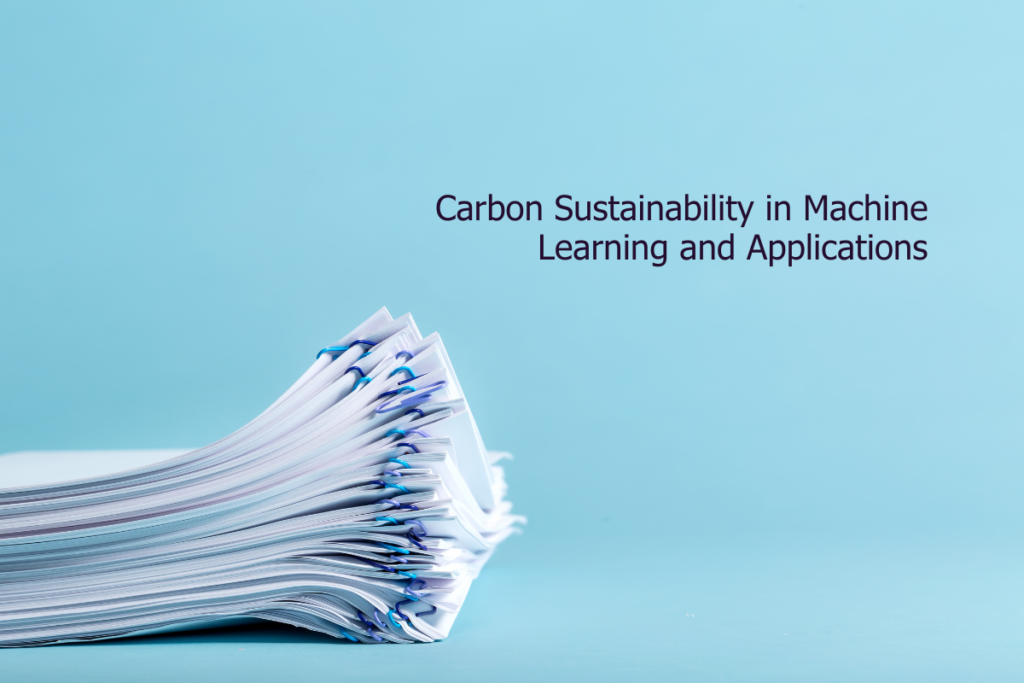
Scope In (Patterson et al, 2021) focuses on the environmental impact of large-scale neural network training, especially in terms of energy consumption and carbon emissions. With the exponential growth of computational demands in training AI models, particularly neural networks, the energy required has increased dramatically. As AI/ML systems evolve, the environmental footprint associated with them […]